Originally published on EEWeb.com on January 17, 2019.
There is still a lot of hype around the AIoT, so it’s important to be able to separate what is currently feasible from what still lies in the future
Buzzwords such as the internet of things (IoT), edge computing, and artificial intelligence (AI) have been circulating for quite some time. They tend to be thrown around quite liberally and can come across as somewhat nebulous to the average reader.
This is because the hype around these words tends to blow the concept out of proportion with descriptions over-selling the technology behind it. This is sad, as there is actually true value behind the terminology! This value can be shown through smart city examples and other embedded concepts that demonstrate how the different technologies can be tied together. However, explaining real-life AI can be complicated as it exists as a system with many interconnected components rather than just a device loaded with some smart software.
This phenomenon of rapidly spreading buzzwords that fills any nook and cranny of related media and technology sites is actually quite common and can be explained through Gartner’s Hype Cycle. Before we get into that, however, let us first define the relevant terminology pertinent to this article:
The internet of things (IoT)
The internet of things is a long-lasting buzzword that simply refers to the trend of “things” being interconnected through a network (usually the internet). The “things,” in this case, do not strictly refer to separate electronic devices; they can also refer to things like clothing (as in wearable electronics) or even people that are using pacemakers and similar devices. Basically, it is every application that can transfer data within a network in some manner.
Edge computing
The original concept of the IoT required data to be sent to the cloud to undergo processing and analysis. However, as the number of devices increases exponentially, many applications have reached a roadblock in which the amount of data transmitted back and forth causes latency issues. By analyzing data at the edge, the device can determine by itself what needs to be pre-processed and/or sent to the cloud and what can be filtered out. Simply said, edge computing just means moving computational power from a central location out to the “edge,” where the internet meets and interfaces with the real world; i.e., the location data is actually gathered.
Artificial intelligence (AI)
AI, as the term is used today, is still located within the concept of a “Narrow AI.” This refers to a program or system able to perform a set of specific tasks without any direct human input on how to do so, as opposed to “General AI,” which is the scary type that we are more familiar with through science fiction. A current example of Narrow AI is text, picture, and speech recognition achieved through machine learning. Such an AI system has gone through thousands, if not millions, of different pieces of data and learned how to differentiate between different inputs. But no matter how sophisticated its predictions become, it is still limited to this narrow scope of tasks.
Smart city
The term “smart city” can be thought of as implementing the above-mentioned concepts with other information and communication technology with the express goal of increasing quality of life in cities. This is achieved through optimizing resource consumption, traffic flow, public security, and so on.
AIoT = AI + IoT
Simply put, the AIoT is the intersection where AI and the IoT meet. This can be thought of as AI moving closer to the edge and letting a larger piece of the computational cake take place where the IoT device is located. Picture a surveillance system that runs facial recognition. Instead of sending any footage to the cloud for analysis, which will cause delays, the data is directly analyzed by the local AI device.
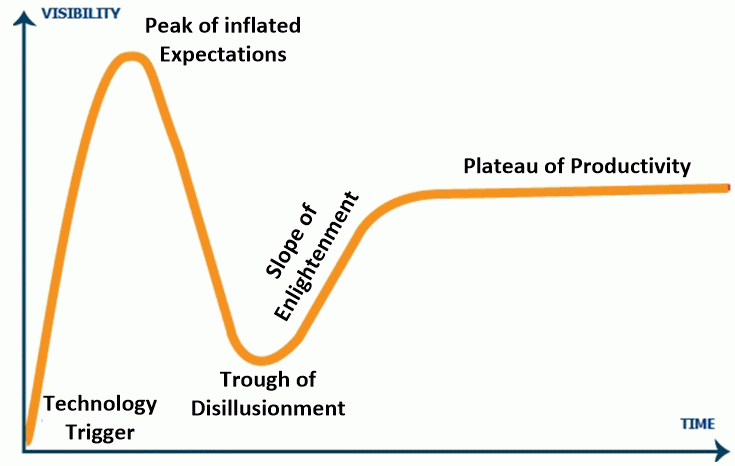
The Gartner Hype Cycle (Source: Innodisk)
AI and IoT on the Gartner Hype Cycle
The Gartner Hype Cycle visualizes the evolution of certain technologies from the initial hype until they eventually reach (or are predicted to reach) maturity and widespread adoption.
Where exactly the terms AI and IoT are located on this curve is hard to pinpoint, not least that it depends on how you define these concepts. However, we are already seeing applications make it onto the market. These applications are not necessarily all that was promised during the initial hype but, rather, toned-down versions. The problem is that truly viable technologies tend to be drowned out by the more hyped applications that are still lingering near the peak of inflated expectations (like quantum computing and self-driving vehicles), but mature AI applications are already climbing the Slope of Enlightenment.
Let us transfer the concepts of IoT and AI into some real-world scenarios, as described below.
Drone traffic monitoring
Our cities are growing in three dimensions by spreading outward and upward (by buildings growing in height). Roads, however, are still mostly confined to two dimensions, which leads to increased traffic congestion as the city grows larger.
Monitoring and altering traffic flow based on real-time data can significantly increase efficiency and cut down congestion. Drones can be quickly deployed to cover large areas simultaneously. By arranging these devices in smart drone configurations, the drones can transfer data to edge devices throughout the city via wireless gigabit communication. By these means, information can be gathered in real time with the drones and then sent off for in-depth analysis by a device located nearby.

Drone with wireless gigabit transmitter (Source: Millitronic)
The first-step analysis is handled by the edge AI platform. This includes vehicle recognition and traffic flow assessment. The device can, thus, determine by itself how to handle the data based on the analysis; i.e., is the number of vehicles increasing and is there a risk of congestion? Any essential data can then be sent to a centralized platform (or the cloud, if you will), where measures such as redirecting traffic, altering speed limits, and adjusting traffic lights can be taken based on the data.
AI platforms and all-flash arrays
As the majority of data processing will still take place in the cloud and edge computing becomes more and more widespread, IoT devices have greater computing power, but network bandwidth is still limited.

AI platform (Source: Aetina)
Real-time analysis can be greatly sped up using an all-flash array as part of the infrastructure to meet the requirements of AIoT workloads.
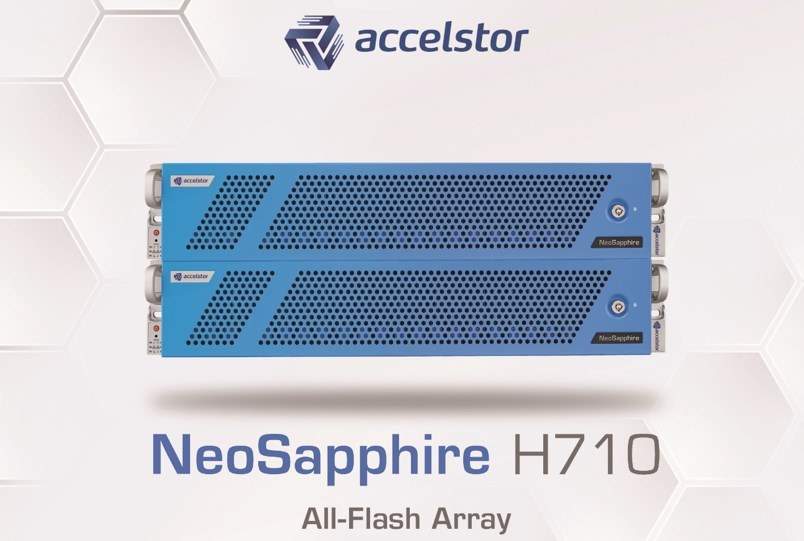
All-flash array (Source: AccelStor)
When footage arrives from the many AI platforms scattered around the city, the all-flash array is instrumental in accelerating data processing speeds for both structured and unstructured data. As this server board is fully loaded with flash devices, it can rack up performance many times faster than by using the more traditional hard disk drives (HDDs).
Fleet management and AI
Keeping with the vehicular theme, AI can also strengthen fleet management operations. Keeping tabs on a large fleet of vehicles can be challenging, but there are many ways to optimize operations: reducing fuel costs, mitigating unsafe driver behavior, vehicle maintenance, and so on.
Most of the current vehicle positioning systems are heavily reliant on GPS, which can have problems. For example, you might have experienced entering a tunnel and having your GPS completely lose track of where you are. This can also happen in cities when driving inside parking garages or other areas with poor satellite coverage. It is also hard for the system to determine the vehicle’s elevation.
However, there are sources of data other than GPS that can give us a pointer on where the vehicles are. For instance, a vehicle’s speed and turning rate can be constantly tracked by the vehicle itself. An onboard AI platform can then calculate where the vehicle is at any moment in time by having these parameters compensate for missing or incomplete GPS data. This technology is called automotive dead reckoning, or DR. Lastly, data can be transmitted through wireless networks back to the operator.
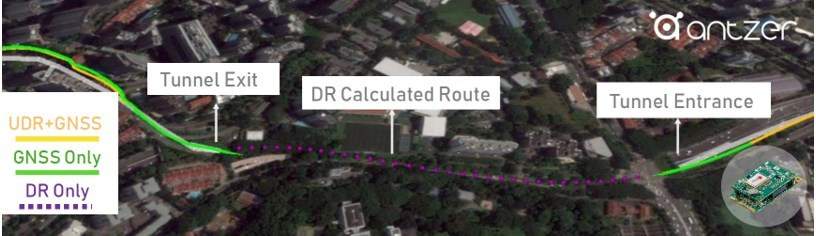
DR allowing for vehicle tracking inside tunnels (Source: ANTZER TECH)
This is how the use of AI devices can help the operator keep track of where vehicles are, even when hidden from the gaze of satellites. Picture this scenario: An accident with one of the vehicles happens inside a tunnel. With just a basic GPS, the operator will not know that an accident has taken place before they are contacted in some way. With an AI solution, on the other hand, the operator will be notified straight away that something is wrong as the vehicle is no longer moving, the engine has been suddenly turned off, etc.
Storage and memory: back to basics
A lot of the talk around the AIoT focuses on bringing the AI to the place where data is gathered. In other words, we need devices that can run these programs out on the road, up in the sky, inside a factory, or on an offshore platform. What these locations have in common are environments that are typically not too favorable to electronic equipment.
This is why it is wrong to think of AI and IoT as a magic box that you buy and install wherever you need it. It should be seen as a system made up of many smaller components that, together, enable the AI to do its work and for data to reach its user without too much delay.
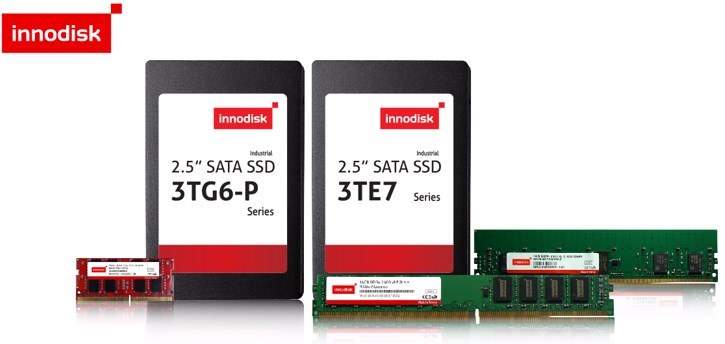
Industrial-grade memory and storage (Source: Innodisk)
Storage and memory components and devices have to be present at every point where data is processed and transferred. As these processes are not usually the most taxing on the system, it is more important that the components are robust and built to last.
Conclusion
There is still a lot of hype around the AIoT, so the important thing when evaluating this technology is being able to separate what is currently feasible from what still lies in the future.
However, if you are able to see the past the buzzwords, there are nuggets of value already available that can enhance our personal lives and businesses.